Unsupervised Pattern Discovery In Automotive Time Series

Abstract
The advent of connected vehicles and the proliferation of sensors has led to an explosion of automotive time series data. This wealth of data holds immense potential for uncovering hidden patterns and trends that can improve vehicle safety, performance, and efficiency. Unsupervised pattern discovery techniques play a crucial role in this endeavor, enabling the extraction of valuable insights from unlabeled data.
5 out of 5
Language | : | English |
File size | : | 26941 KB |
Text-to-Speech | : | Enabled |
Screen Reader | : | Supported |
Enhanced typesetting | : | Enabled |
Print length | : | 236 pages |
Hardcover | : | 134 pages |
Item Weight | : | 10.2 ounces |
Dimensions | : | 6.14 x 0.38 x 9.21 inches |
Automotive time series data is a complex and multifaceted collection of measurements collected from various sensors embedded in vehicles. These measurements include engine performance parameters, fuel consumption, tire pressure, and a myriad of other variables. Analyzing this data presents a significant challenge due to its high dimensionality, noise, and non-stationary nature.
Unsupervised pattern discovery techniques offer a powerful approach to address these challenges. Unlike supervised learning methods that require labeled data, unsupervised techniques can identify patterns and structures in unlabeled data. This makes them particularly well-suited for automotive applications where labeled data may be scarce or expensive to obtain.
Unsupervised Pattern Discovery Techniques
A wide range of unsupervised pattern discovery techniques have been developed for analyzing automotive time series data. These techniques can be broadly categorized into two main approaches: clustering and dimensionality reduction.
Clustering
Clustering algorithms group similar data points together, forming clusters. This process can help identify distinct patterns or behaviors in the data. Common clustering algorithms used in automotive applications include k-means, hierarchical clustering, and density-based spatial clustering of applications with noise (DBSCAN).
Dimensionality Reduction
Dimensionality reduction techniques aim to reduce the number of features in the data while preserving the most important information. This can make the data more manageable and easier to analyze. Principal component analysis (PCA) and singular value decomposition (SVD) are popular dimensionality reduction algorithms used in automotive time series analysis.
Applications of Unsupervised Pattern Discovery in Automotive
Unsupervised pattern discovery has a wide range of applications in the automotive industry, including:
- Anomaly detection: Identifying unusual or unexpected patterns in vehicle data can help detect potential faults or malfunctions.
- Predictive maintenance: Analyzing time series data can help predict component failures and schedule maintenance accordingly, reducing downtime and improving vehicle safety.
- Vehicle characterization: Clustering techniques can be used to group vehicles based on their performance characteristics, aiding in vehicle design and optimization.
- Driving behavior analysis: Unsupervised pattern discovery can uncover patterns in driving behavior, such as aggressive driving or fuel-efficient driving habits.
Case Study: Anomaly Detection
To illustrate the application of unsupervised pattern discovery in automotive, let's consider the case of anomaly detection. By analyzing time series data from a fleet of vehicles, we can identify patterns that deviate from normal operating conditions. These deviations may indicate potential issues that require attention.
Figure 1 shows an example of anomaly detection using k-means clustering. The data points are clustered into two groups: normal operating conditions (blue) and anomalous conditions (red). The anomalous cluster represents a small subset of data that deviates from the majority of the data.
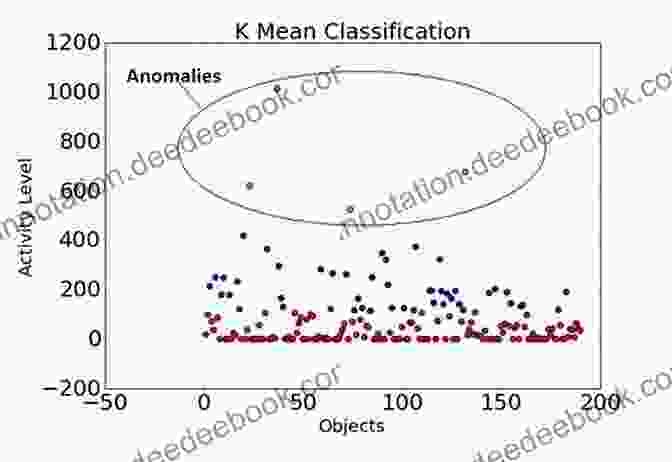
Unsupervised pattern discovery is a powerful tool for extracting valuable insights from automotive time series data. By identifying patterns and structures in unlabeled data, these techniques offer a valuable approach to improve vehicle safety, performance, and efficiency. As the volume and complexity of automotive data continues to grow, unsupervised pattern discovery will play an increasingly important role in the future of automotive engineering and transportation.
5 out of 5
Language | : | English |
File size | : | 26941 KB |
Text-to-Speech | : | Enabled |
Screen Reader | : | Supported |
Enhanced typesetting | : | Enabled |
Print length | : | 236 pages |
Hardcover | : | 134 pages |
Item Weight | : | 10.2 ounces |
Dimensions | : | 6.14 x 0.38 x 9.21 inches |
Do you want to contribute by writing guest posts on this blog?
Please contact us and send us a resume of previous articles that you have written.
Book
Page
Chapter
Text
Genre
Library
Paperback
E-book
Newspaper
Paragraph
Bookmark
Glossary
Bibliography
Preface
Synopsis
Annotation
Footnote
Scroll
Codex
Tome
Library card
Memoir
Narrator
Character
Librarian
Card Catalog
Borrowing
Archives
Research
Lending
Reserve
Academic
Reading Room
Rare Books
Special Collections
Literacy
Study Group
Thesis
Storytelling
Theory
Cynthia Go
Betho Ieesus
Andrea Angelini
Dennis Eugene Dey
Michael Nelson
Anne E Mcmills
Steve Jenkins
John B Manbeck
Bart Plantenga
Sally Ablett
Nancy Belfer
Lauren Sandler
Jean Lau Chin
Nick Louth
Paul Stevens
Carolyn Cohagan
Maryjo Koch
Phillip Brown
Timothy E Clinton
Christy Davis
Light bulbAdvertise smarter! Our strategic ad space ensures maximum exposure. Reserve your spot today!
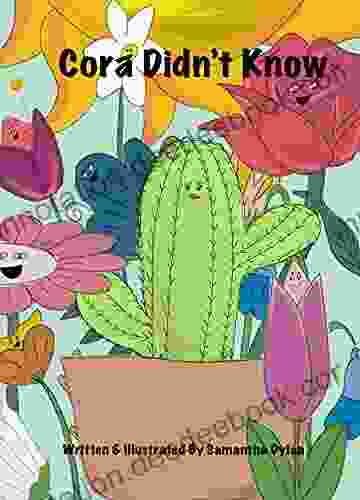

- Aleksandr PushkinFollow ·7.6k
- Evan HayesFollow ·17.6k
- Pat MitchellFollow ·18.3k
- Dalton FosterFollow ·10k
- Natsume SōsekiFollow ·11.5k
- Oliver FosterFollow ·17.6k
- Adrien BlairFollow ·18.7k
- Morris CarterFollow ·19k
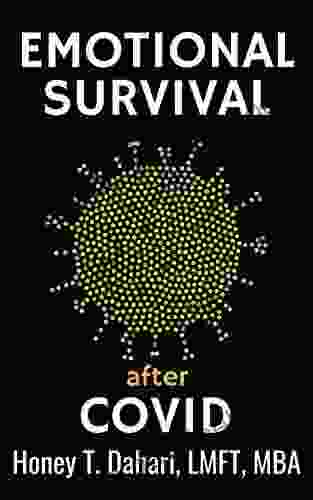

Your Mental Health and Wellness in the Post-Pandemic Era:...
The COVID-19 pandemic has...
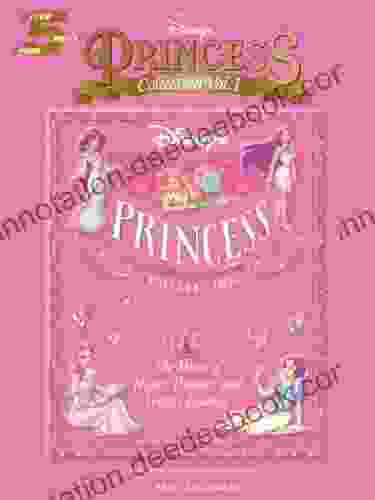

The Music of Hope, Dreams, and Happy Endings: Five-Finger...
In the realm of beautiful music, there...
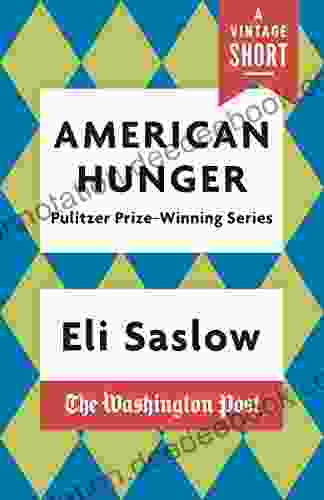

The Pulitzer Prize-Winning Washington Post Vintage Short:...
The Washington Post Vintage Short, an...
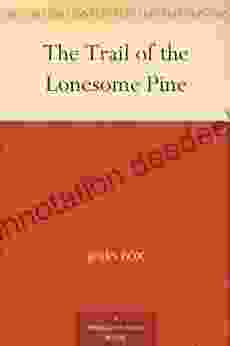

The Trail of the Lonesome Pine: A Majestic Journey into...
Nestled amidst the...
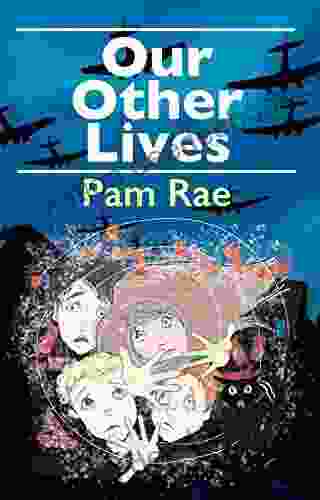

Our Other Lives by Christina Geist: Exploring the...
Our Other Lives by Christina Geist is a...
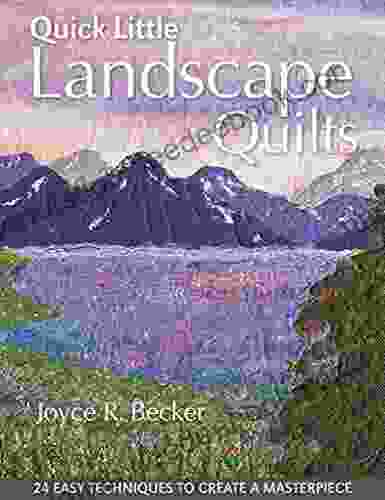

24 Easy Techniques to Create a Masterpiece
Creating a...
5 out of 5
Language | : | English |
File size | : | 26941 KB |
Text-to-Speech | : | Enabled |
Screen Reader | : | Supported |
Enhanced typesetting | : | Enabled |
Print length | : | 236 pages |
Hardcover | : | 134 pages |
Item Weight | : | 10.2 ounces |
Dimensions | : | 6.14 x 0.38 x 9.21 inches |